Nonlinear kernels, dominance, and envirotyping data increase the accuracy of genome-based prediction in multi-environment trials
Von einem Mystery-Man-Autor
Last updated 12 Juni 2024
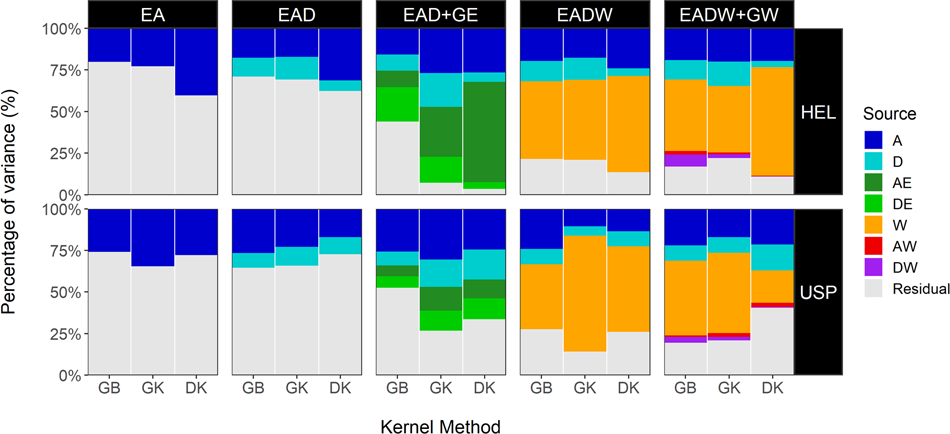
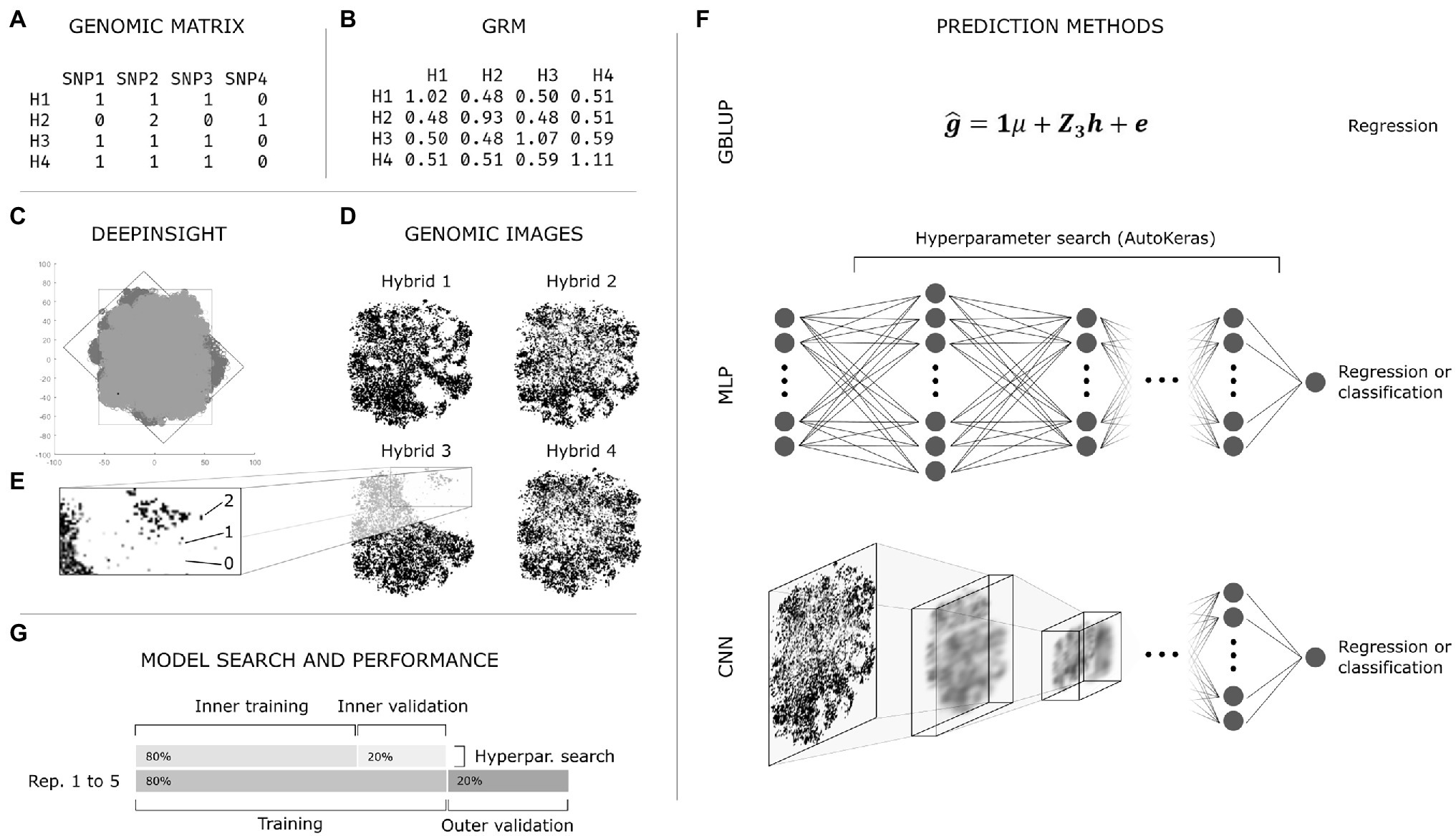
Frontiers Automated Machine Learning: A Case Study of Genomic “Image-Based” Prediction in Maize Hybrids
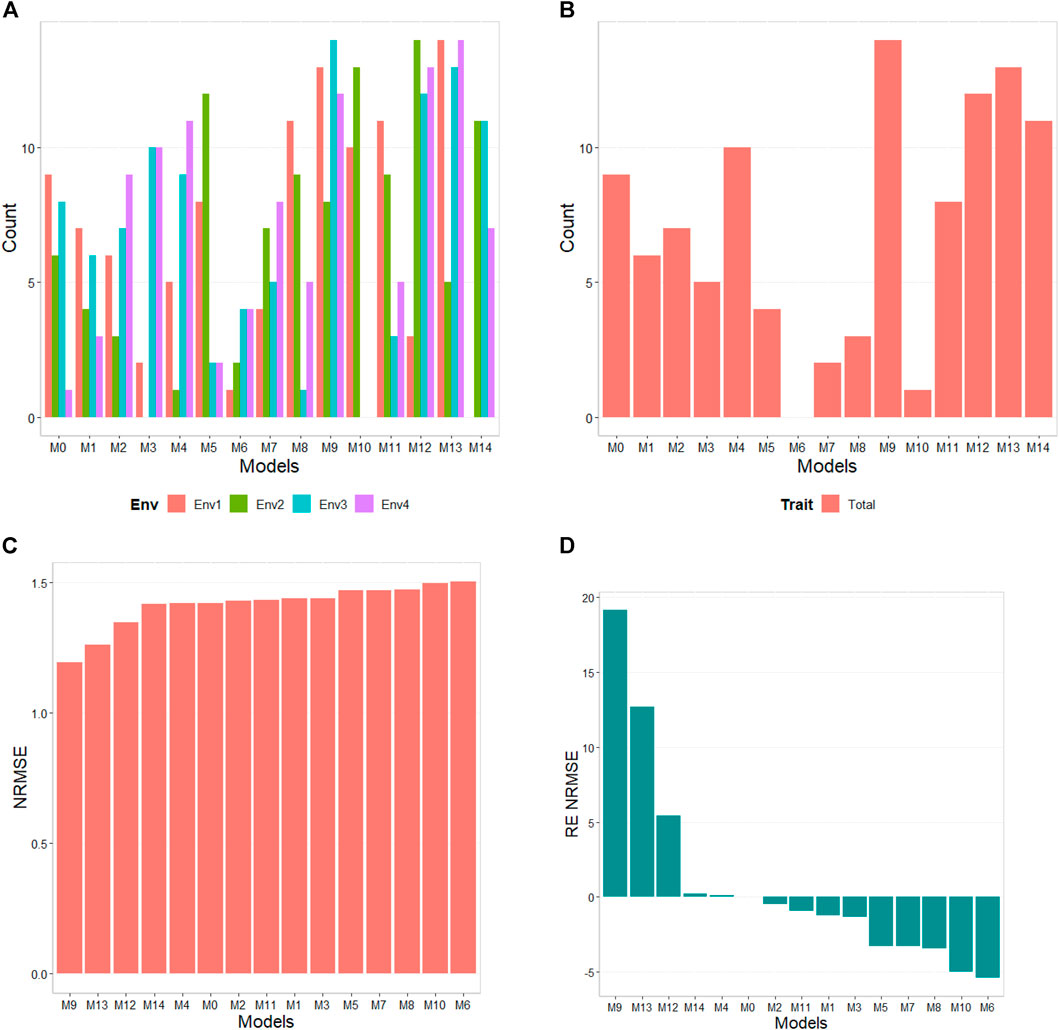
Frontiers Do feature selection methods for selecting environmental covariables enhance genomic prediction accuracy?

PDF) Nonlinear kernels, dominance, and envirotyping data increase the accuracy of genome-based prediction in multi-environment trials

Weighted Kernels Improve Multi-Environment Genomic Prediction
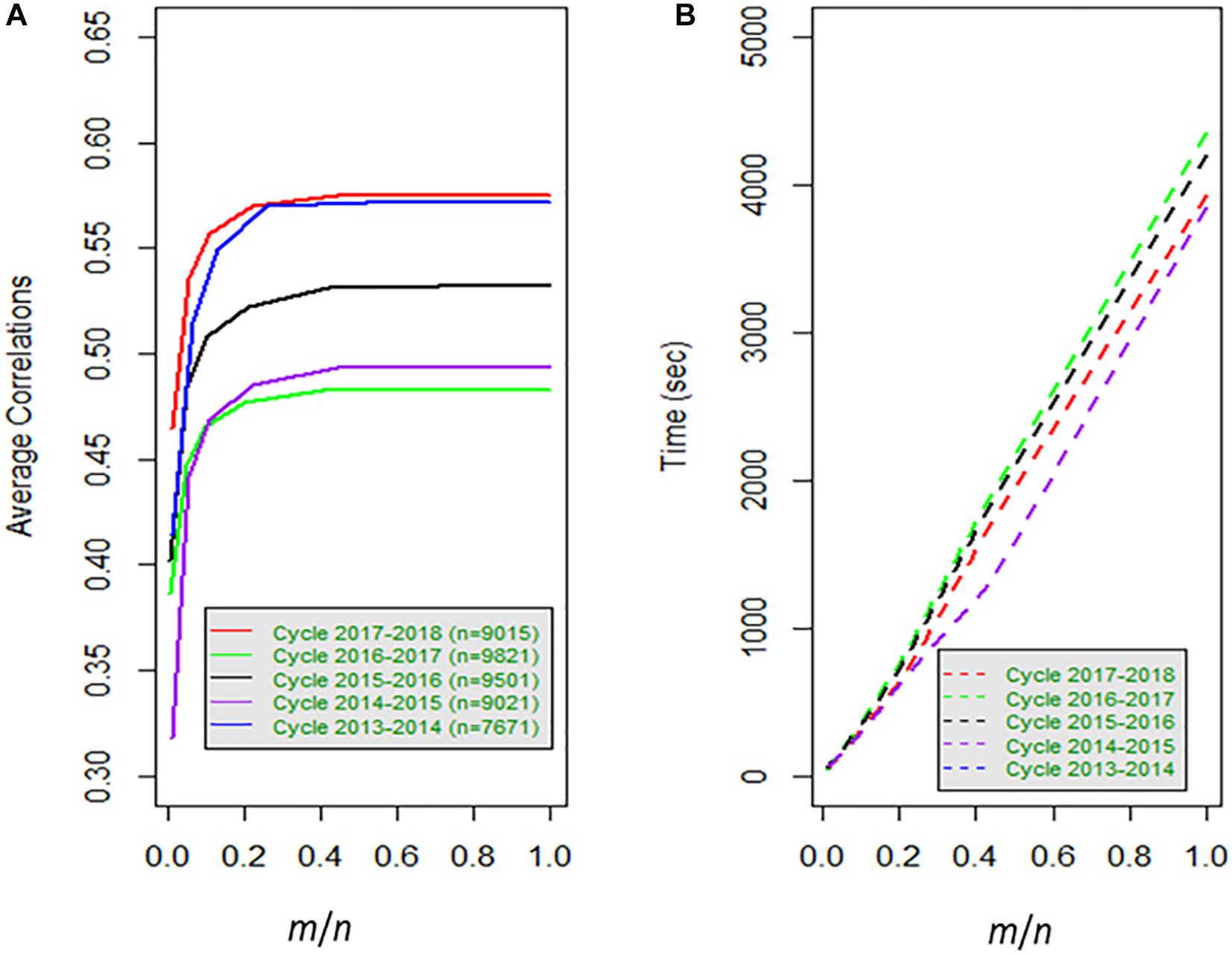
Frontiers Approximate Genome-Based Kernel Models for Large Data Sets Including Main Effects and Interactions
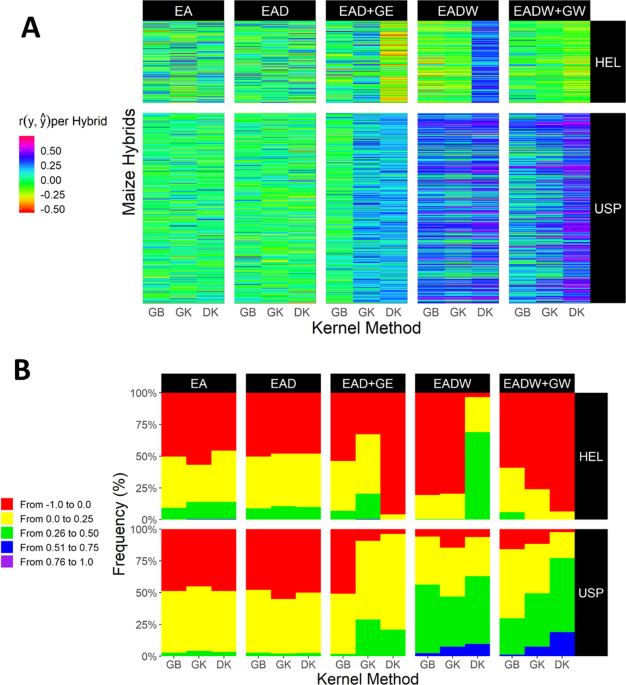
Nonlinear kernels, dominance, and envirotyping data increase the accuracy of genome-based prediction in multi-environment trials

Nonlinear kernels, dominance, and envirotyping data increase the accuracy of genome-based prediction in multi-environment trials
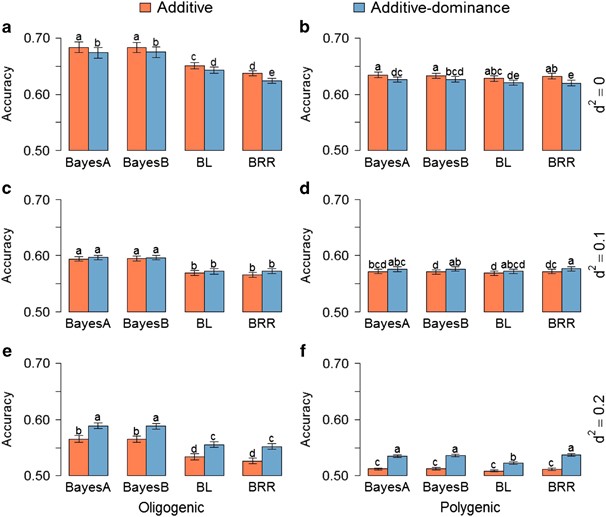
The contribution of dominance to phenotype prediction in a pine breeding and simulated population
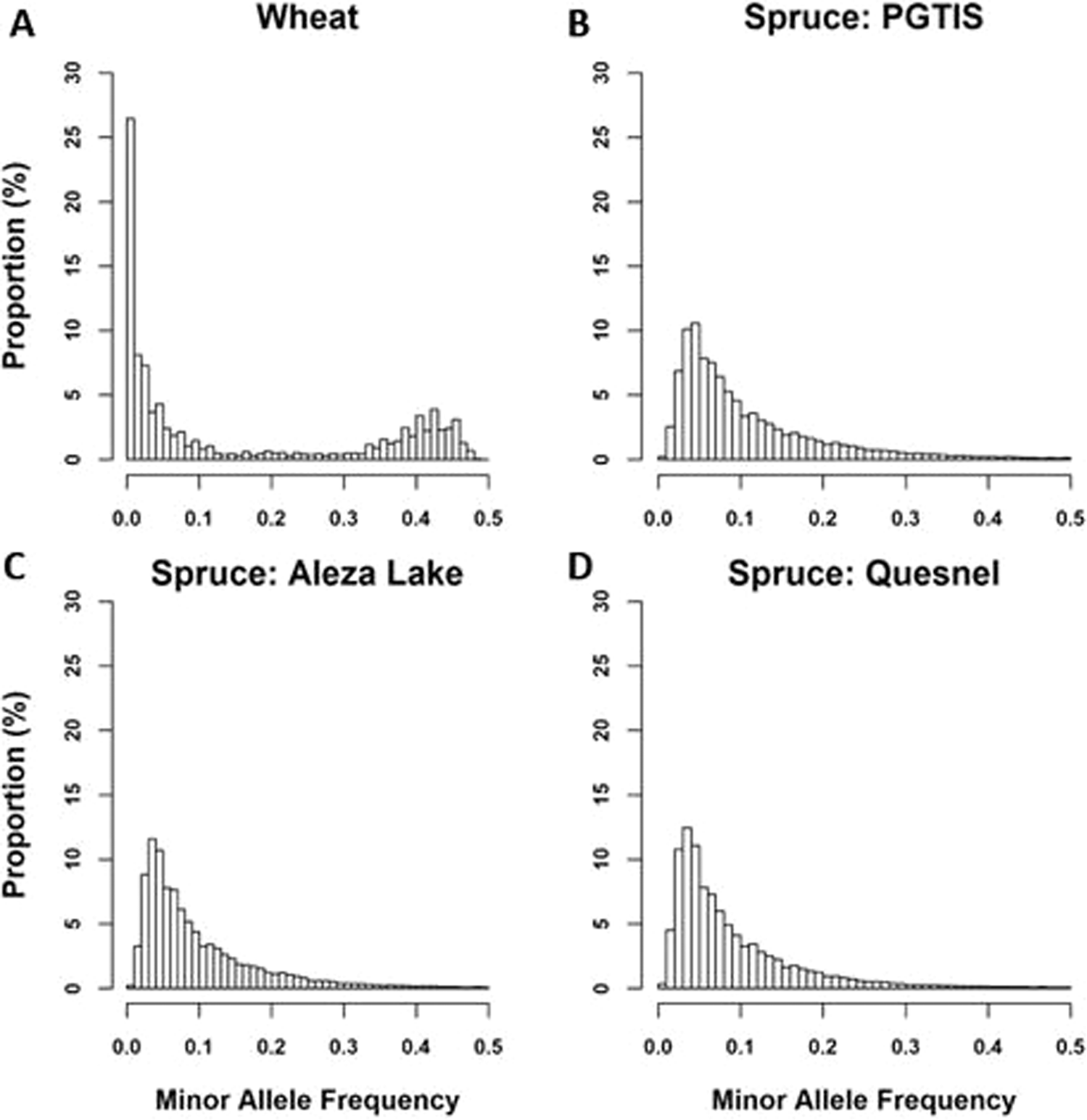
Weighted kernels improve multi-environment genomic prediction
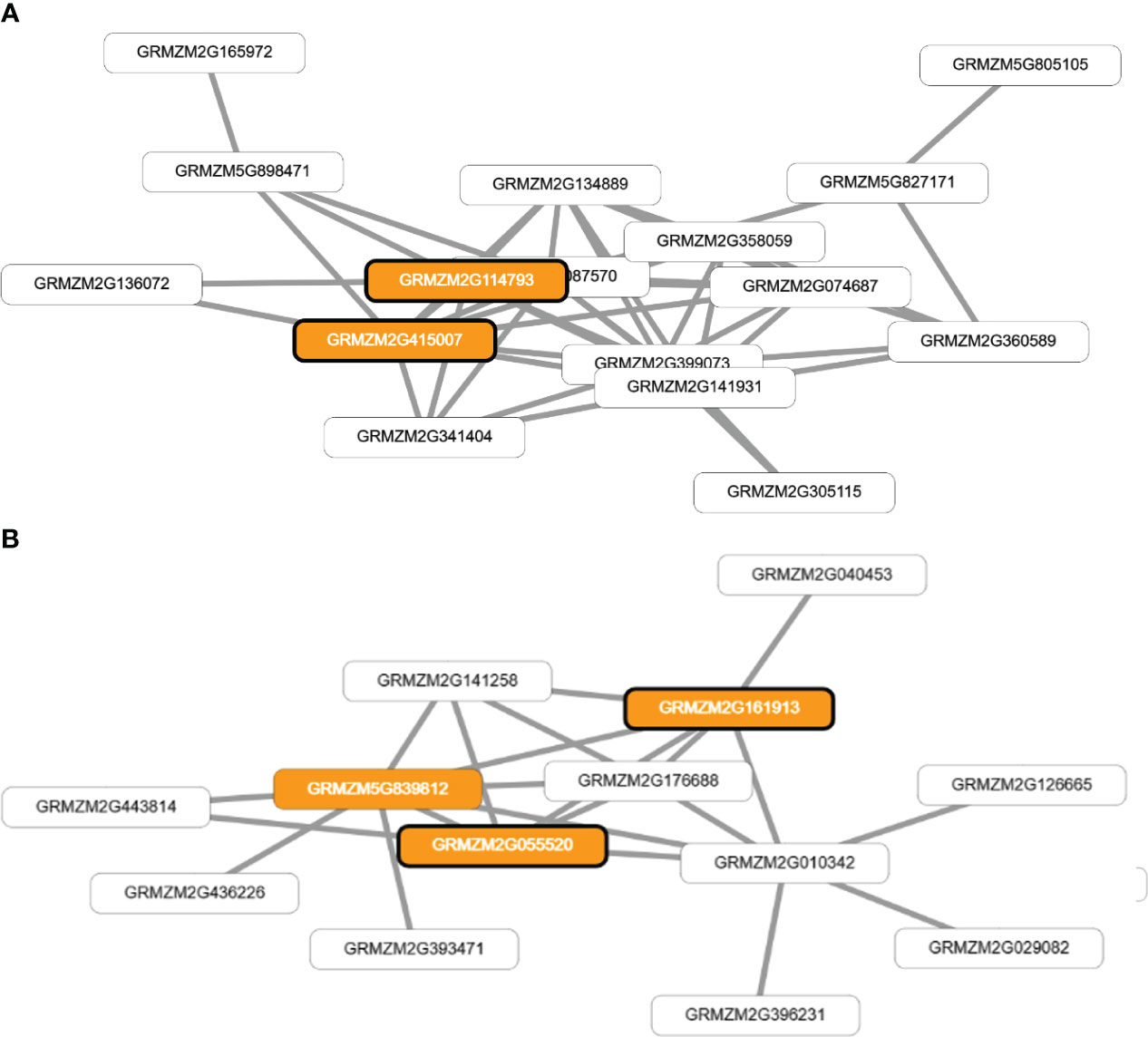
Frontiers Multi-trait and multi-environment genomic prediction for flowering traits in maize: a deep learning approach
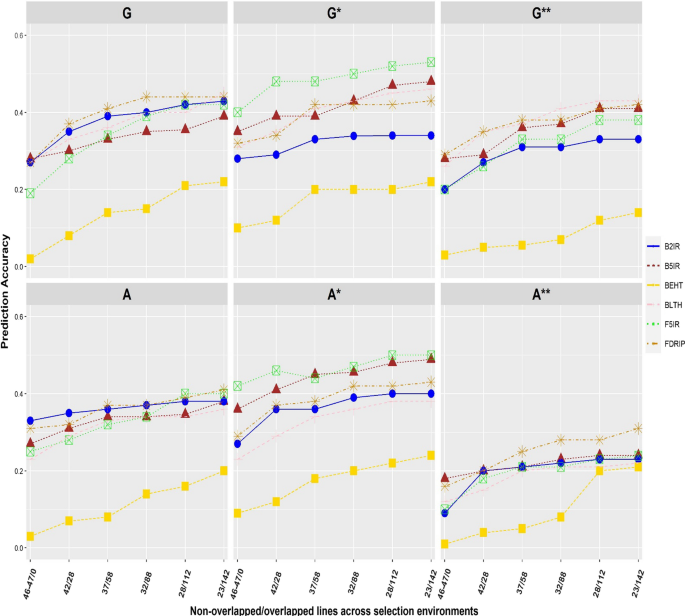
Sparse testing using genomic prediction improves selection for breeding targets in elite spring wheat
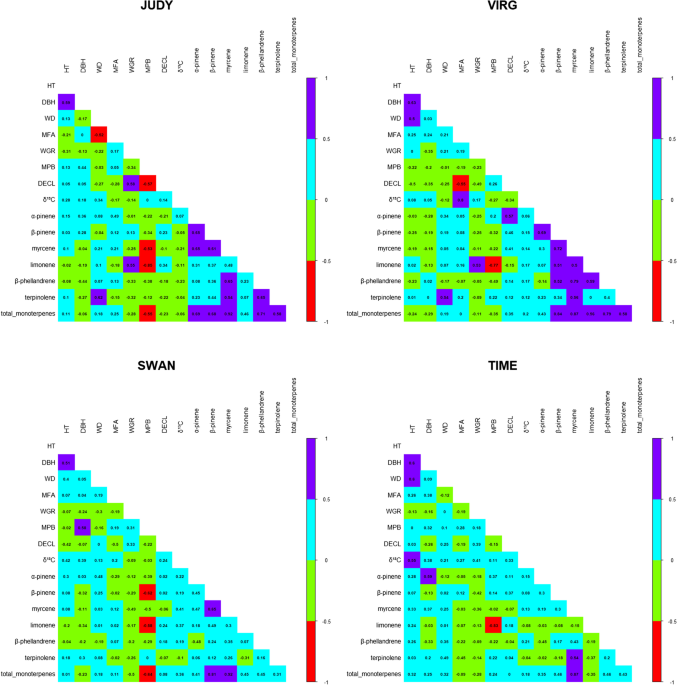
Multiple-trait analyses improved the accuracy of genomic prediction and the power of genome-wide association of productivity and climate change-adaptive traits in lodgepole pine, BMC Genomics
für dich empfohlen
- GK R 1/160/40G-FSTF14 Jul 2023
- Genie Wireless Keyless Entry Keypad (GK-R) – The Genie Company14 Jul 2023
- Most see him rollin', some be hatin': The Rota GKR14 Jul 2023
- Führungswagen THK THK - ref. HSR-15-R-SS (CK-GK) - RUBIX Deutschland14 Jul 2023
- BOS Umfassungszarge GK125 Bud3 1-teilig BRM: 625x2125mm L/R für GK-Wand14 Jul 2023
- Zugkupplung Albe Berndes EM 80-R Ausf. GK , Gesamtgewicht 800 kg , Zugmaul . Kugelkupplung , Zugrohr rund 70 mm14 Jul 2023
- GKR Group Manufacturer of Plastic Injection Moulds14 Jul 2023
- Top 600 General Knowledge Questions and Answers PDF Download: Enhance Your GK Knowledge with GKelite14 Jul 2023
- ASONPAO GK-R/GWKPD-BL Auto-Seek 315/390MHZ Keyless Entry Wireless Keypad 37224R for IntelliCode Genie Garage Door Opener(1Pack)14 Jul 2023
- CM6321-R 12V DC 30A STARTER RELAY FOR GO KART GK 200D, F, H, E & MORE *OEM*14 Jul 2023
Sie können auch mögen
- 3Pcs Auto Tür Fensterheber Schalter Taste Abdeckung Gehäuse Innen Dekoration Zubehör Für Benz Smart Fortwo Forfour 451 453 - AliExpress14 Jul 2023
- orig. ANDRIS® Marken-Piktogramm Sicherheits-Aushang Schild „Verhalten bei Unfällen“, Erste Hilfe, Notfall, Hinweis, Betriebsaushang, ISO-Kunststoffplatte 200x200 mm14 Jul 2023
- Shop PSM Style Carbon Fiber Trunk Lip Spoiler Online14 Jul 2023
- BGS Ventilfederspanner Ventilfeder Spannapparat Ventil Federspanner 35 - 200 mm14 Jul 2023
- LEICKE Blocker-Karten-Set RFID Karte(für Universal meisten Kreditkarten (VISA, Mastercard), EC-Karten, Scheckkarten, Führerschein- und Mitgliedskarten, Türschloss-Karten, Personalausweise, Reisepässe, Chipkarten, Zugangskarte, Bankkarten)14 Jul 2023
- Magnetplatten - Buchbindermeister2414 Jul 2023
- PopMount 2 Auto und Tisch Schwarz Halterung14 Jul 2023
- Caliber Audio Technology RMD120DAB-BT-B Autoradio Bluetooth MP314 Jul 2023
- Hazet Universal Kugelgelenk-Abzieher 1790-714 Jul 2023
- Silikon Hülle kompatibel mit Apple iPhone 13 Mini Case transparent Handyhülle Cars Disney Pixar Lightning McQueen 95: : Elektronik & Foto14 Jul 2023